Michael Scherbela
I’m a machine learning researcher with a background in physics and management consulting. I enjoy technology, working with great people, and being outdoors.
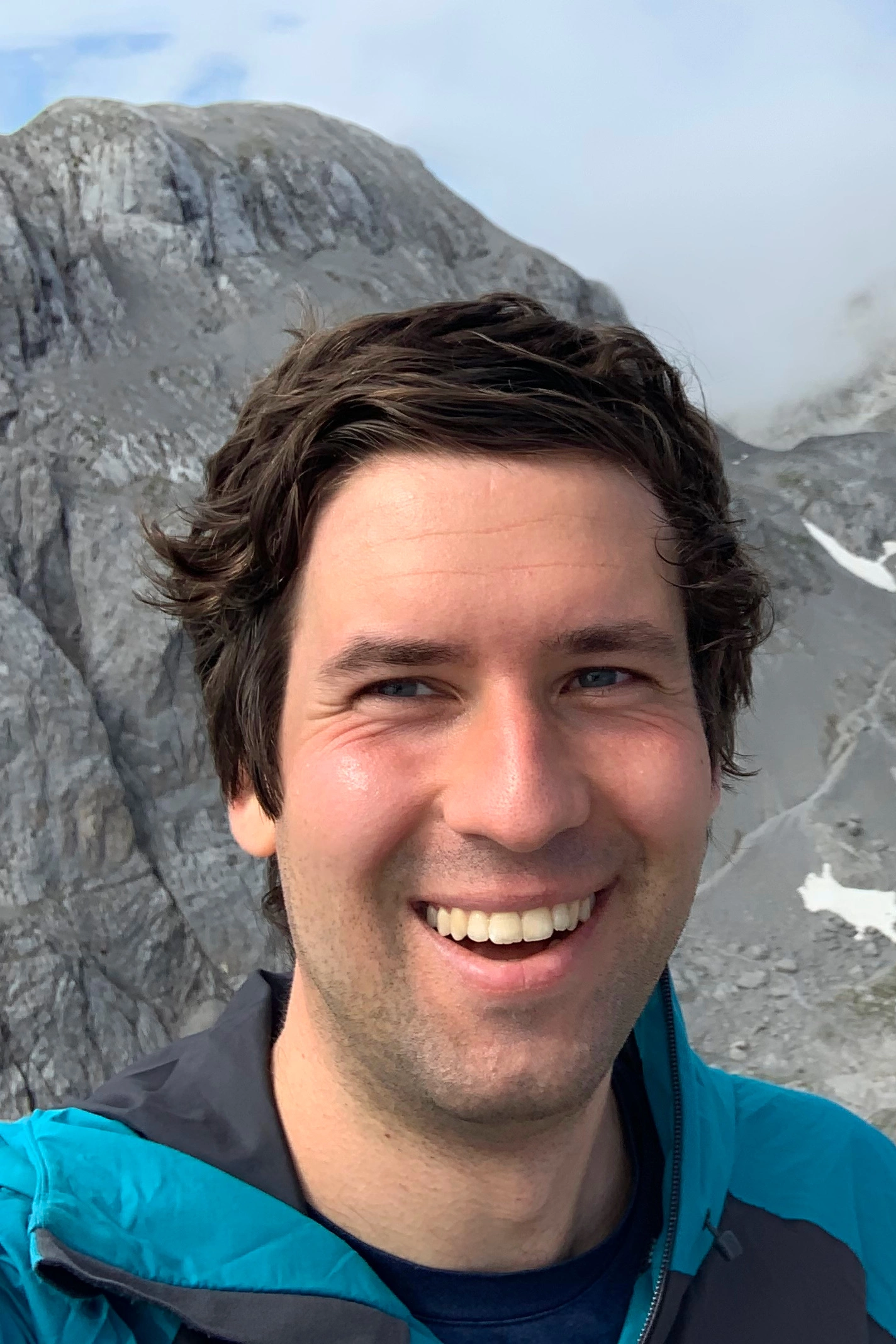
Education and Research
University of Vienna
· Vienna, AustriaPhD: Mathematics / Machine Learning
Using deep-learning to develop better ab-initio methods for quantum chemistry. This is my thesis on Transferable Neural Network Wavefunctions
Graz University of Technology
· Graz, AustriaMaster: Physics
Studying physics with a focus on computational methods. Master thesis on using machine learning to predict surface structures of molecules adsorbing to metal surfaces. Graduated with distinction.
Graz University of Technology
· Graz, AustriaBachelor: Physics
Studying physics and graduating with a bachelor thesis on using Matrix Product States to simulate quantum spin systems. Graduated with distinction.
Work Experience
McKinsey & Co
· EuropeConsultant
Top-management consulting for a diverse set of clients across Europe, focusing on data- and technology-driven projects
Virtual Vehicle Research Center
· Graz, AustriaStudent Researcher
Experimental and computational research to improve automotive systems
Infineon Technologies
· Graz, AustriaSummer Internship
Automating testing of semiconductor devices during development
SLR Engineering Technologies
· Graz, AustriaWorking Student
Software development for image recognition in traffic control applications
Anton Paar
· Graz, AustriaSummer Internship
Assembly of high precision density measurement devices
Research Highlights
I work on the intersection of machine learning and quantum chemistry. A full and up to date list of my publications can be found on Google Scholar .
Scientific papers
Year | Title | Authors | Journal |
---|---|---|---|
2025 | Accurate Ab-initio Neural-network Solutions to Large-Scale Electronic Structure Problems | Scherbela, Gao, Grohs, Günnemann | arxiv |
2024 | Transferable Neural Wavefunctions for Solids | Gerard, Scherbela, Sutterud, Foulkes, Grohs | arxiv |
2023 | Variational Monte Carlo on a Budget - Fine-tuning pre-trained Neural Wavefunctions | Scherbela, Gerard, Grohs | NeurIPS 2023 |
2023 | Towards a Transferable Fermionic Neural Wavefunction for Molecules | Scherbela, Gerard, Grohs | Nature Communications |
2022 | Solving the electronic Schrödinger equation for multiple nuclear geometries with weight-sharing deep neural networks | Scherbela, Reisenhofer, Gerard, Grohs, Marquetand | Nature Computational Science |
2022 | Gold-standard solutions to the Schrödinger equation using deep learning: How much physics do we need? | Gerard, Scherbela, Grohs, Marquetand | NeurIPS 2022 |
2020 | Charge Transfer into Organic Thin Films: A Deeper Insight through Machine‐Learning‐Assisted Structure Search | Egger, Hörmann, Jeindl, Scherbela, Obersteiner, Todorovic, Rinke, Hofmann | Advanced Science |
2019 | SAMPLE: Surface structure search enabled by coarse graining and statistical learning | Hörmann, Jeindl, Egger, Scherbela, Hofmann | Computer Physics Communications |
2018 | Charting the energy landscape of metal/organic interfaces via machine learning | Scherbela, Hörmann, Jeindl, Obersteiner, Hofmann | Physical Review Materials |
2017 | Structure prediction for surface-induced phases of organic monolayers: overcoming the combinatorial bottleneck | Obersteiner, Scherbela, Hörmann, Wegner, Hofmann | Nano Letters |
Presentations
Year | Type | Title | Venue | Link |
---|---|---|---|---|
2022 | Invited Talk | High accuracy wavefunctions using deep-learning-based variational Monte Carlo | IPAM, UC Los Angeles | Recording on youtube |
2022 | Summer School Tutorial | Basics of Machine Learning | Erwin Schrödinger Institute, Vienna | Material on GitHub |
2021 | Poster Presentation | Sharing is Caring: Accurate ab-initio wavefunctions through weight-sharing neural networks | EPFL, Lausanne | Corresponding paper |
2017 | Talk | Structure Search using Machine Learning | DPG Spring Meeting, Dresden | |
2017 | Talk | Structure Search at Interfaces using Bayesian Regression | IMPRESS Conference 2017, Helsinki |
Personal projects
I enjoy working on small side projects. Some of them are silly, but I’ve learned a lot from all of them. Here are a few which I particularly enjoy.